Machine learning reveals new findings for lung disease
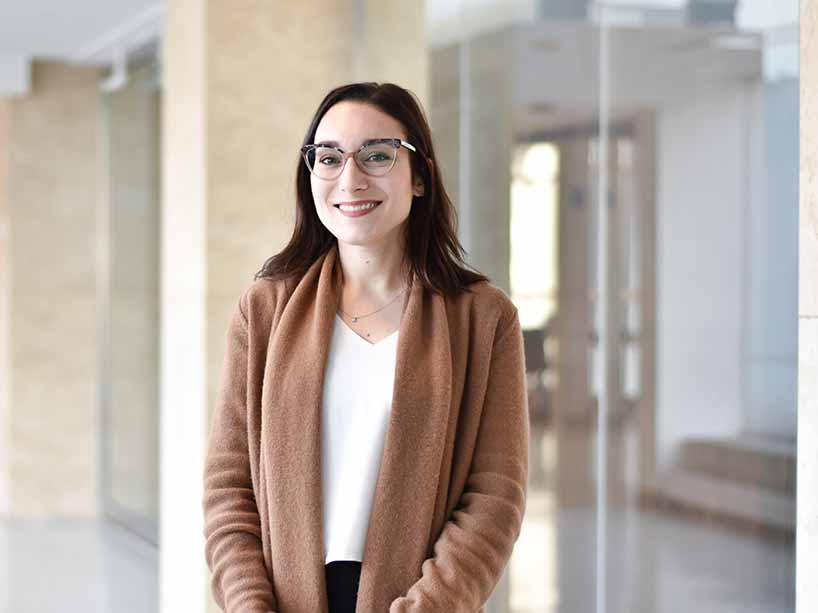
Miranda Kirby was named a Canada Research Chair in 2019 and has been focused on studying how to extract new information from quantitative medical imaging to understand lung disease in a deeper way. Photo by Sarah McIntyre.
Since being named Canada Research Chair (CRC) in June 2019, physics professor Miranda Kirby has taken an interdisciplinary approach to her research on lung disease, including cystic fibrosis and smoking-related lung disease. Her team develops new methods that allow them to learn more about the underlying disease in the lung, where it is located and how severe it is – information that is not available with conventional clinical tools.
Kirby’s research focus is quantitative medical imaging and she’s now studying how to extract new information from images to understand lung disease in a deeper way. To do so, she has teamed with some collaborators to combine medical imaging, like CT scanning, with machine learning.
“Specifically, these methods allow us to quantify the underlying disease pathologies, to gain a much deeper understanding of how the disease initiates in the lung and progresses over time,” Kirby says.
Her interest in the intersection of science and math started as early as her undergraduate degree when she completed a double major in applied math and biology. “At the time, that was considered a unique combination of disciplines,” she says. “I was always really interested in quantitative sciences, such as math, but I was also interested in studying anatomy and physiology and understanding how things work.”
Her graduate studies in medical biophysics took this interdisciplinary approach to the next level. “Imaging aligns well with medical biophysics research, which is really a perfect marriage between physics, math and the application of studying the human body.”
Machine learning
Once these medical images have been collected from research participants, Kirby says her team is able to apply quantitative methods to the images to unlock more information. That’s because there’s more information in CT scans than perhaps meets the eye.
“Radiologists assess certain features in these images, like whether emphysema is visible or not. But with quantitative methods, we can say how much emphysema is there, how clustered it is in the lung, where it’s distributed, and numerous other ways of characterizing the disease. Then with machine learning, we can determine which of those features of the disease we quantify are important, so we don’t need to guess.”
Her lab is extracting information that people like radiologists can visually identify but in very quick, standardized and reproducible ways. To do so, they’ve developed computer code that’s able to process the images and extract numbers in a way that the team can then interpret.
“We know that disease in the airways is important to disease pathogenesis and patient outcomes, so we come up with ways of quantifying what we’re seeing based on our understanding of disease, and based on what our pathologists and respirologists collaborators tell us is important,” says Kirby.
“We then figure out ways of assigning quantitative values, which gives us a new measurement of disease. We might have an outcome, like hospitalizations that we would like to predict - we want to know which patients are at risk of being hospitalized. We collaborate with other researchers at Ryerson and other institutions to conduct machine learning, which learns how to identify patients that will have future hospitalizations, based on those measurements.”
The path ahead
Since her CRC appointment, Kirby has been focusing on training her seven graduate students and expanding the scope of her research by building new collaborative relationships. These relationships have taken her down new and exciting avenues, including research on cannabis smoking and vaping.
“With the legalization of cannabis, lots of us in the research community that study lung disease, particularly smoking-related lung disease, have been really interested in what the effect of cannabis use will be on long-term lung health,” she says. “This really gives us the opportunity to tease it apart from tobacco smoking. Now we have the opportunity to prospectively acquire data and really answer the question.”
Kirby says that this research will have impact as far as knowledge generation goes. “The real outcome is being able to inform the public on what impact smoking cannabis may have on their lung health so they can make more informed decisions.” Kirby hopes to expand this research to study the effects of vaping as well.
And down the road, she says she’s hoping to translate everything that she’s doing to low-dose imaging or imaging that does not use ionizing radiation imaging, like MRI. “A focus on lung structure traditionally requires imaging modalities like CT that provide really useful information, but the flipside is that it exposes patients to greater risk because of the ionizing radiation. Once we have developed structural measurements for low-dose CT imaging or no-dose imaging like MRI, then there’s the possibility to move our research methods into a pediatric group, studying kids and infants, so we can use these measurements to better manage lung disease throughout the lifespan.”